Client Satisfaction Prediction Model
Jan
2023
Ongoing
Problem / Purpose
The company needed a way to proactively identify clients at risk of dissatisfaction or termination, and to highlight those likely to provide referrals. Existing feedback (e.g., NPS) came too late for proactive action.
Solution
Built an end-to-end ML pipeline using Salesforce data to predict client satisfaction as Delighted, Satisfied, or Poor. Engineered ~300 features capturing client profile (size, industry, tenure), communication patterns (volume, direct vs. employee, sentiment via Snowflake Cortex, time to resolve), risk indicators (AR balance, layoffs, internal handoffs), product usage, referral history, and summarized NPS trends. Trained a LightGBM model in DataRobot, prioritized top 16 features, handled class imbalance, and automated scoring/prediction flow (Snowflake ↔ DataRobot ↔ Salesforce). Developed feedback loop for future feature enhancement.
Key Achievements / Impact
Enabled weekly satisfaction prediction to proactively engage at-risk clients and strengthen referral outreach. Improved visibility into client health, supported client success workflows, and provided a foundation for ongoing model refinement.
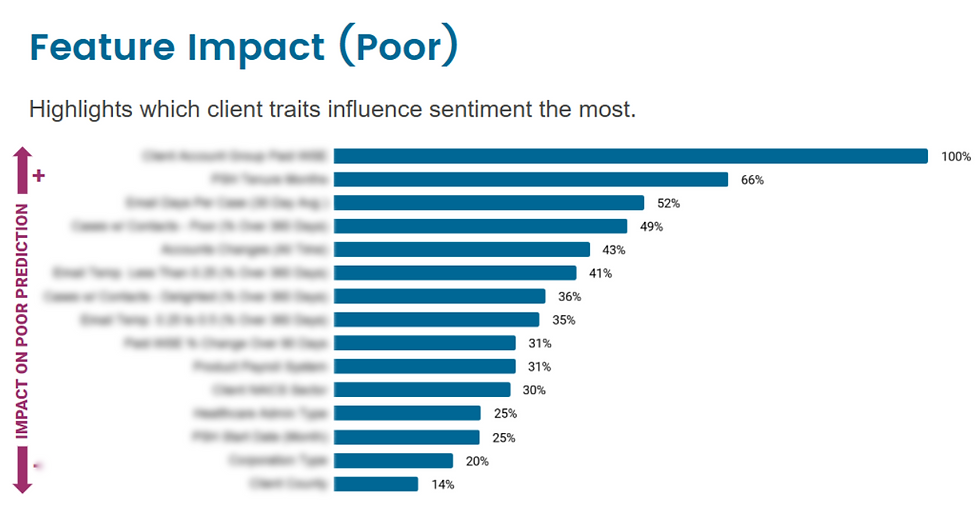

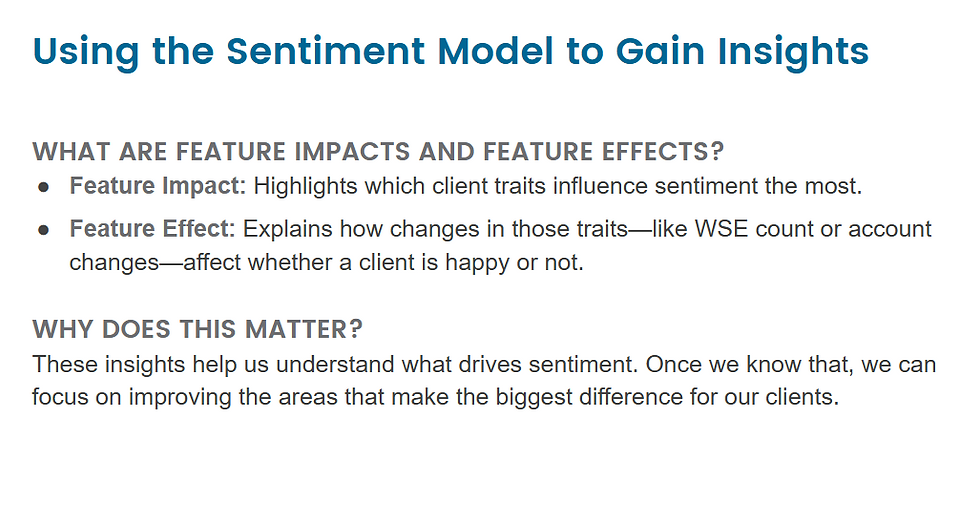
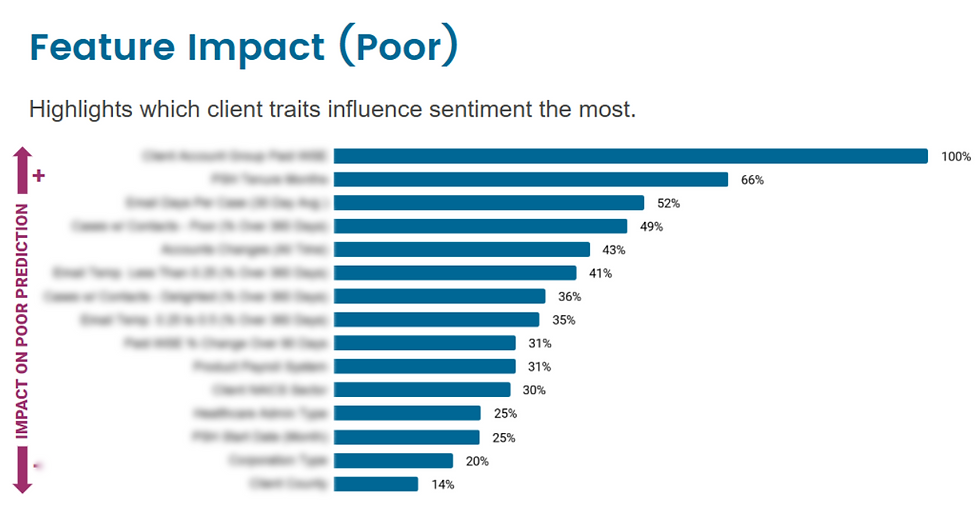
Key Technologies / Tools Used
DataRobot, Snowflake, Salesforce, ML Models (Classifier), Feature Engineering, Class Imbalance Handling, End-to-End Data Pipeline Design, SQL
Role
Data Scientist
ProService Hawaii